We must not limit artificial intelligence through our own flawed thinking
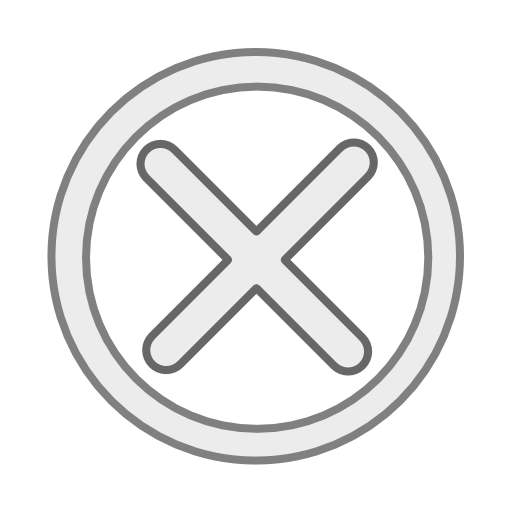
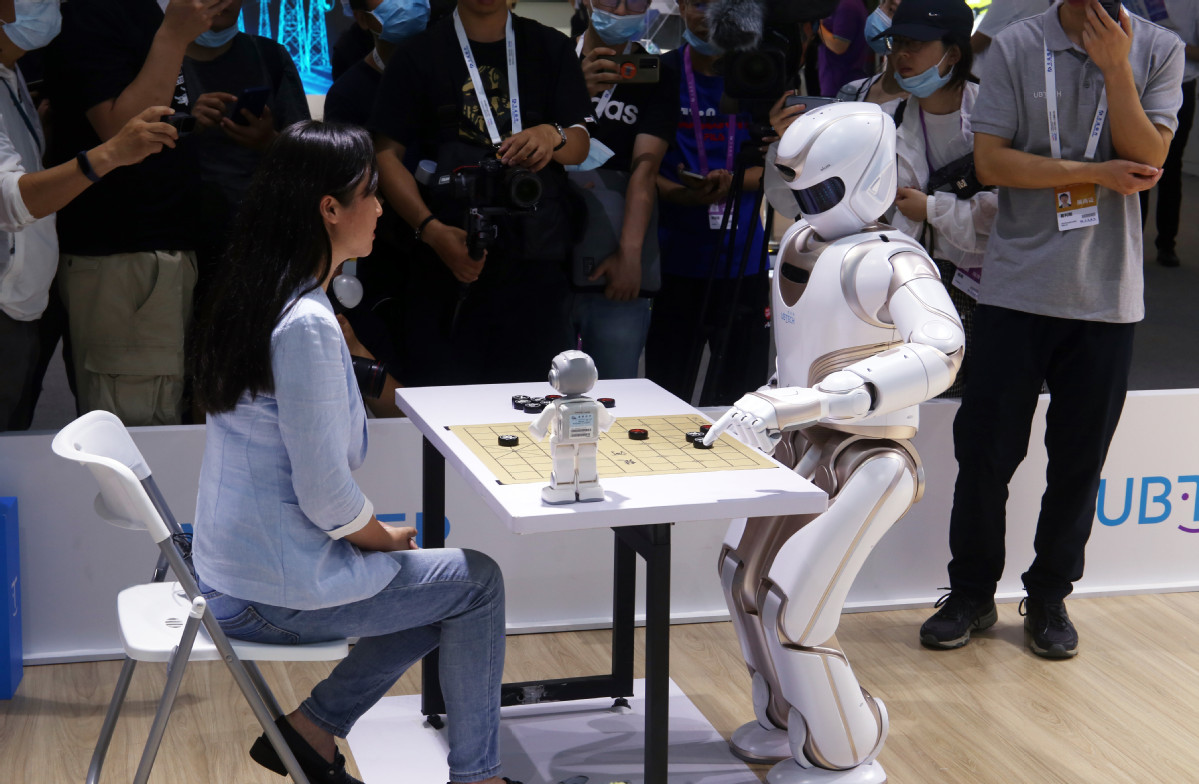
Artificial intelligence has given us insight into the complex challenges we face in our technical age. But it is vital that researchers are careful in ensuring that AI develops in a way which is not limited by human design flaws and thinking.
This is not just a philosophical discussion; there are real practical implications for the world's most powerful technologies to become dangerously homogenized in operating the same way. Undermining the very premise of what makes AI so promising can only be prevented through careful developmental review and strong academic foundation.
Developers, while hard working and creative, do not wish to waste time reinventing the wheel. When coding clever AI programs to perform certain tasks, they often base them upon what are called "foundation" AI models. Such AI programs are frighteningly smart, and can crunch through giant quantities of data in whatever task they have been purposed for.
Their overuse, however, can have negative consequences. Any single point of failure in the system will be inherited model to model down the line, like a genetic defect in a living organism, but with no chance of beneficial mutation.
In 2019, developers at Google built an AI program which now plays a role in nearly all of Google's search functions. Called BERT (Bidirectional Encoder Representations from Transformers), it is a natural language model that was adopted by many other big tech companies who did little other than add new functions to the basic codes.
Such practices are commonplace where profit and quick results are prioritized. How practical this remains in the long term, however, is questionable. The industry is aware of the hole it is digging itself, but research environments will only see real change when perspectives are switched from a purely revenue-based standpoint.
Before 2019, foundational models were not mainstream. Previously, researchers designed bespoke AI models for tasks ranging from document organizers to virtual assistants, which had a diverse spectrum of designs and thinking patterns, arguably leading to a healthier AI ecosystem.
Deep-rooted biases are already starting to appear in these foundational models and, if allowed to continue, may affect the way we trust how AI thinks and operates in years to come on. Studies published in the New York Times indicated that BERT tended to associate the word "programmer" with men rather than women, a generalization that may have knock-on effects for any applications based on BERT's code.
Ensuring that ethics teams have a cross-disciplinary background in areas such as philosophy, politics, law, history and science, is vital in ensuring that foundational AI systems remain in tune with reality, if they are to be used.
The danger remains, however, that such systems will not be subject to regular scrutiny, if the assumption is that identical models have been checked historically, and are assumed to be ready to go.
Studies throughout modern psychology's century-long history show that groups of humans are more susceptible to flawed thinking and overlooked errors if diversity in said groups is low.
Solutions are often found more rapidly when teams have a variety of viewpoints, creativity and analytical skills. Transferring this concept to AI has an immediate and powerful urgency, given how much we now rely on the technology's ability to think for us.
Meanwhile the consequences will be suffered all too vividly by us alone.
- Innovative products, new tech highlighted at 2021 ZGC Forum
- G20 conference on AI, robotics calls for increased public-private collaboration
- AI reshaping health and wellness sector
- AI gadgets, solutions on show at World AI Conference in Shanghai
- AI tech promising to make urban lives smarter and better